We closed the loop with #DIYPS and now have a DIY closed loop artificial pancreas running right now (for me, aka n=1.)
Here’s what a closed loop #DIYPS artificial pancreas looks like:
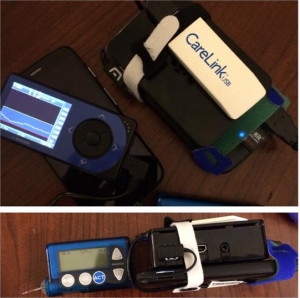
(A raspberry pi powered with a battery (shown) or via an outlet; a Carelink USB plugged into the Pi and in close proximity to the pump; a Medtronic insulin pump (an older version, thanks to a family from CGM in the Cloud for sharing it!); and of course my CGM and Android uploader phone that’s sending my data into the cloud/#DIYPS)
Here’s how a closed loop artificial pancreas or “#DIYPS closed loop” works:
Over the past year, we’ve been using #DIYPS to process BG, insulin on board and carbohydrate decay in real time. This yields real-time predictive alerts of three kinds: more insulin needed (X.X units of bolus insulin); less insulin needed (temp basal to zero insulin for X minutes); or carbs needed. Using this algorithm and my data entered (insulin taken or reduced; carbs ingested); we’ve seen significant results not only for the first 100 days, but also sustained over an entire year for improved average glucose, improved a1c, and improved time in range.
Closing the loop with #DIYPS
Three weeks ago, we mentioned at D-Data Exchange 2014 that we were planning to close the loop with #DIYPS…by August 1 (our wedding date :)).
The FDA was even in the room.
Two weeks later…we closed the loop.
It was this simple:
- Thanks to Ben West’s awesome work, we plugged the Carelink USB into the Raspberry Pi. Thanks to a generous family from the CGM in the Cloud Facebook group, we acquired an old 522 insulin pump that could talk to the Carelink USB. (My current pump is too modern to work with this setup.)
- Once we established that the Pi/USB setup could read from the pump, we started connecting #DIYPS to the system to close the loop.
- Normally, #DIYPS provides 3 types of recommendations (see above). We reconfigured it so that while “looping”, it only enables the pump to do temporary basal rates instead of boluses. If #DIYPS says I need more insulin, it tells the pump to begin a temporary basal rate for 30 minutes above my normal basal rate. If #DIYPS says I need less insulin, it tells the pump to begin a temporary basal rate lower than my normal basal rate for 30 minutes, effectively reducing my net insulin.
- When something changes and I need to do more or less insulin, #DIYPS tells the pump to increase or decrease the temporary basal rate, or resume my normal basal rate.
- Note: there is a maximum basal rate that the pump can provide. This is a safety check so that #DIYPS cannot recommend a seriously dangerous basal rate. In fact, if I need more insulin than #DIYPS closed loop can safely provide using a 30 minute temp basal, the system will alarm and alert me to that state (although I usually already know about it). Same for if I was dropping fast and needed carbs in addition to reducing insulin; it would alarm and recommend carbs above and beyond reducing insulin.
I’ve been ‘looping’ (using #DIYPS closed loop) for a little over a week. Here are some of my overnight results:
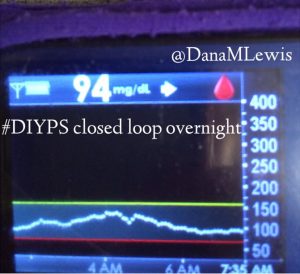
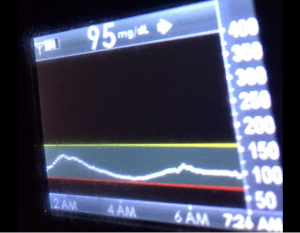
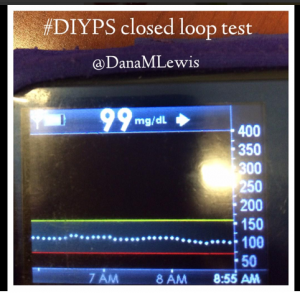
As you can see, the outcomes are awesome. In fact, they are better than I expected!
#DIYPS closed loop results are significant
Here’s a break down that shows on an example night (Sunday night, 12/14 through Monday morning, 12/15) of how #DIYPS performed:
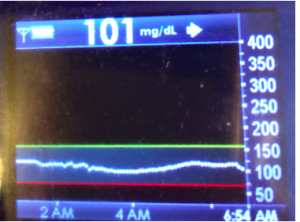
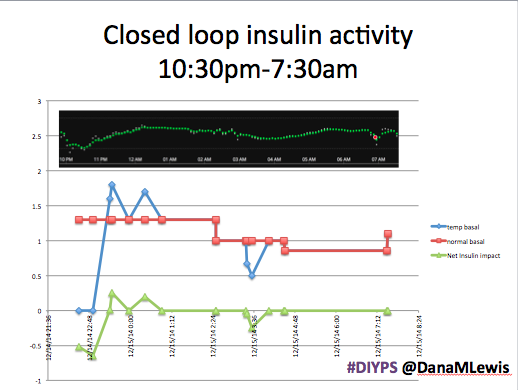
You can see that around 10:30 (not pictured, but in the graph), I was running low and needed some temp basals to zero, then later some higher temp basals to accommodate the resulting rise in BG. Later, my normal basal rates sufficed until another point overnight when I needed less insulin to prevent a low.
While this is 1/9 or so example nights, this is pretty much how it works every night. BGs drift up? Temp basal higher (usually 1.6 or 1.8, which is <=.5u above my normal). BGs drifting low? Temp basal lower or in extreme cases to zero to prevent a low.
What happens if the closed loop artificial pancreas fails/stops working?
If the loop stops working*, #DIYPS continues to work normally (processing all data and pushing recommendations), and my pump goes back to my normal basal profiles after any existing temp basal rates expire (which would be a max of 30 minutes). No big deal and no more dangerous than I would be walking around with my pump acting normally.
*I’ve found numerous ways to make the system stop working: no battery power; being too far away from the USB stick so the pump can’t communicate; no cell signal to provide wifi as a hotspot to the Raspberry Pi; if the pump battery goes low, RF gets turned off and the USB can’t talk to the pump; if there’s no cell signal or wifi at all, I can’t upload CGM data to #DIYPS anyway; if the SD card gets corrupted inside the Raspberry Pi; etc.
So, there’s many points of failure. But it doesn’t matter. #DIYPS closed loop is safer for me (disclaimer: just me! I’m the only one doing this as a personal experiment to my own body) than if I just had a pump and a CGM, because it can reduce insulin and prevent lows. And it can increase insulin slightly and prevent rebounds.
After about a week’s worth of data, here’s the results of #DIYPS (what I’ve been using for the past year) versus the week of closed loop #DIYPS:
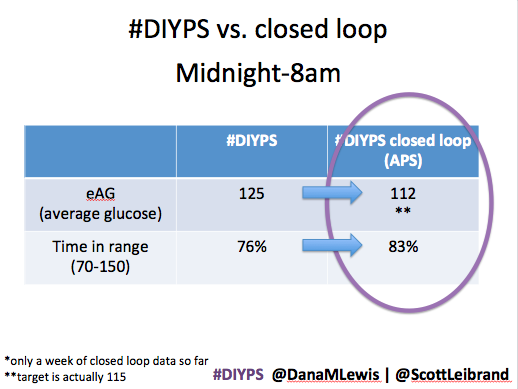
The target BG is especially awesome, because we have the closed loop targeting a BG of 115!
It’s also worth noting the reduction in alarms. Remember, I sleep through CGM alarms, so we built #DIYPS. #DIYPS makes a lot of alarms – sometimes upwards of 40 a night (those are really bad nights!). With the closed loop nights, there are very few alarms..and they’re usually alerts about things #DIYPS closed loop is taking care of on it’s own (see all of those no-hitters above!).
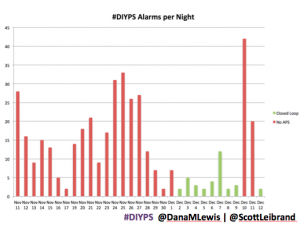
What’s next with #DIYPS closed loop
We’re going to continue and iterate on the closed loop and keep making it better. I’m definitely going to keep using it overnight – it’s like having a working pancreas, the kind where you can go to sleep without fear.
We’re also adding this to the ‘things we’ve learned while working on #DIYPS’ list:
- Calculating the carbohydrate absorption rate for an individual – how many carbohydrates your body absorbs in an hour
- Tracking “carbs on board” or “carb decay” – how many carbohydrates are still being digested in your body and impact BG levels
- “Net” IOB – tracking net IOB by incorporating “negative” boluses from temporary basal rates to zero
- Preventing post-meal BG spikes/drops with early meal boluses and better understanding of insulin activity
- … and you can control overnight BGs solely using simple adjustments of insulin rates.
Thanks to everyone who made it through a really long post, and for the MANY people who have contributed in various ways to helping us close the loop with #DIYPS! Also, let us know what questions come to mind that we haven’t managed to answer above about how the system works, results, etc.
Recent Comments